How AI can help deliver the next generation of RNA medicines
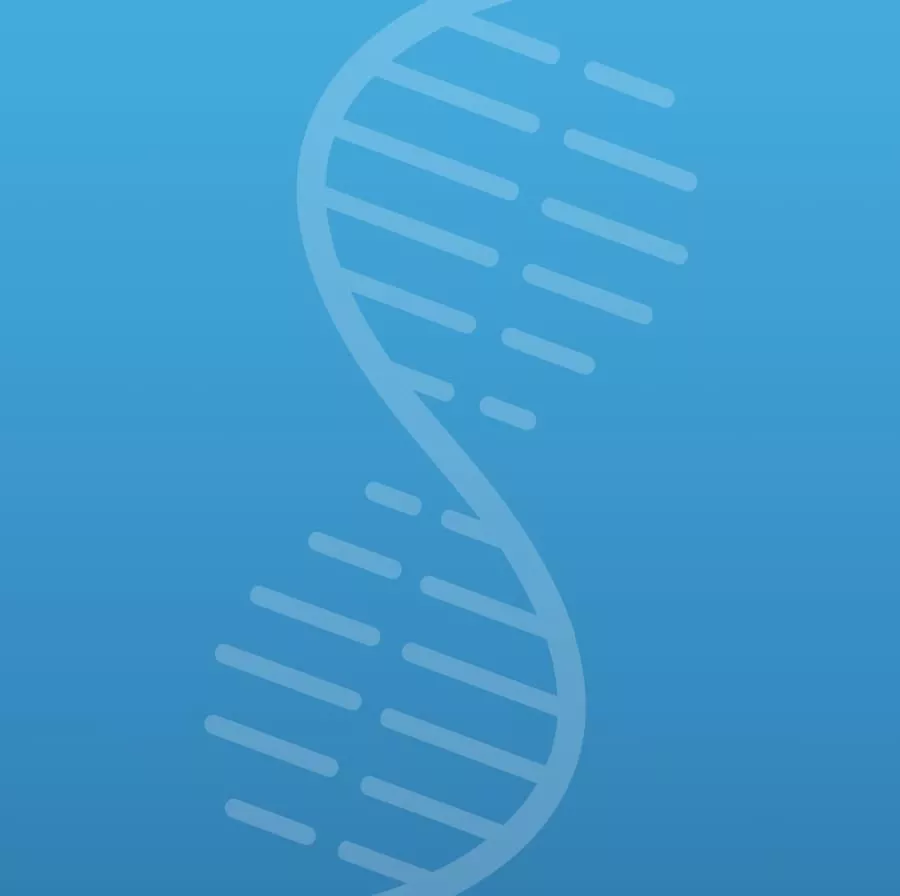
The Inaugural RNA and AI Symposium organized by Sanford Labs explored how advances in AI are accelerating RNA research and therapeutic development, with discussions on AI-driven models for drug discovery, RNA structure, and disease treatment, highlighting AI's growing role in the next generation of RNA medicines.
The 2024 Nobel Prizes highlighted significant recent advances in both AI and RNA biology. David Baker from the University of Washington, along with Demis Hassabis and John Jumper from Google DeepMind, were recognized for developing AI tools to predict protein structures. In addition, Victor Ambros and Gary Ruvkun were honored for their discovery of microRNAs and their fundamental role in health and disease. Notably, this marks the second consecutive year in which a major advance in RNA biology has been recognized: last year, Katalin Karikó and Drew Weissman received Nobel Prizes for their groundbreaking work on mRNA, which was crucial to the development of COVID-19 vaccines.
Sanford Labs recently organized the Inaugural RNA and AI Symposium, aimed at exploring how advances in AI are influencing RNA biology and accelerating the next generation of RNA medicines. Over 150 participants from academia and industry gathered at the Roth Auditorium at the Sanford Consortium for Regenerative Medicine to hear presentations from leading experts and emerging researchers. The symposium was led by the RNA Center for Technologies and Therapeutics at UC San Diego, with Gene Yeo, Professor of Molecular Medicine at UC San Diego and Chief Scientist at Sanford Labs, serving as the host.
As in many other fields, AI is playing an increasingly central role in analyzing large, complex datasets—often revealing insights that are difficult to extract through manual methods. Brendan Frey of DeepGenomics discussed building foundational AI models to identify drug targets and design therapeutic candidates. Yoseph Barash, a faculty member at the University of Pennsylvania, is combining RNA language models with clinical data to identify functional regulatory elements. David Kelley from Calico described a model that predicts cell- and tissue-specific RNA-seq coverage from DNA sequences, shedding light on how cis-regulatory sequences influence mRNA expression. Hani Goodarzi from UCSF is using mostly long-read transcriptomes to build models that predict the relative abundances and structures of mRNA isoforms.
One particularly promising approach is the generation of specialized datasets for training machine learning models. Silvi Rouskin from Harvard developed DMS-MaPseq (dimethyl sulfate mutational profiling with sequencing), a method for assessing RNA secondary structure, and is now using it to train models that explore how RNA structures and RNA-binding proteins regulate viral life cycles. Karen Mei, a Project Scientist in Gene Yeo’s lab, conducted detailed studies on mRNA extracted from neuronal synapses, using these data to train an RNA language model for identifying the rules governing mRNA localization at synapses.
Other researchers are focusing on building more sophisticated structural models—an inherently difficult task, given the limited number of experimentally determined RNA structures, especially those that involve RNA-protein or RNA-small molecule interactions. These efforts often rely on researchers creating tailored datasets for specific challenges. Stephan Eismann, Head of Machine Learning and co-founder of Atomic AI, discussed how they integrate machine learning models with large-scale, in-house wet-lab screens to identify functional binders to RNA targets. Chungwen Liang from Creyon Bio shared how they combine statistical models with physics-based approaches to create more accurate structural models of oligonucleotide-based therapeutics.
Several presentations also highlighted AI’s potential in therapeutic development. Jeff Milton, CEO of La Jolla Labs, described how combining AI with advances in antisense oligonucleotide technology and next-generation sequencing allows for faster design and screening of personalized therapies. Rose Yu, a faculty member at UC San Diego, discussed the use of variational autoencoders to generate drug candidates with specific properties, such as high binding affinity and solubility. Lastly, Huiwen Ju from NVIDIA presented the BioNeMo framework, a collection of tools, libraries, and models designed for computational drug discovery, which can be easily integrated with GPU-based computational resources.
In summary, the symposium underscored AI’s transformative potential in advancing RNA medicine. Machine learning models are increasingly able to identify drug targets, predict key aspects of RNA biology, and guide therapeutic development. With continued innovation, AI is poised to play a crucial role in realizing the promise of RNA-based therapies in the near future.